Data Analytics in Agriculture
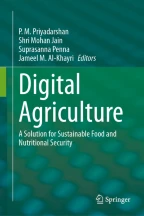
Food security is a crucial global need, threatened by population growth, climate change, and decreasing arable land. Data-driven agriculture is the most promising approach to solving these current and future problems by improving crop yields, reducing costs, and ensuring sustainability. As the number of smart sensors and machines on farms increases and a greater variety of data is used, farms will become increasingly data-driven, enabling the development of smart farming. This is possible, thanks to new technologies that enable massive data storage, such as cloud computing and Hadoop, in addition to processing and analysis through Big Data and machine learning. In this chapter, we explain some practical examples of their use.
This is a preview of subscription content, log in via an institution to check access.
Access this chapter
Subscribe and save
Springer+ Basic
€32.70 /Month
- Get 10 units per month
- Download Article/Chapter or eBook
- 1 Unit = 1 Article or 1 Chapter
- Cancel anytime
Buy Now
Price includes VAT (France)
eBook EUR 181.89 Price includes VAT (France)
Hardcover Book EUR 232.09 Price includes VAT (France)
Tax calculation will be finalised at checkout
Purchases are for personal use only
Similar content being viewed by others
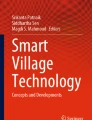
Big Data for Smart Agriculture
Chapter © 2020
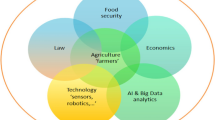
Data analytics for crop management: a big data view
Article Open access 23 December 2022
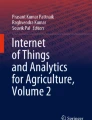
Data Analysis and Prediction Using Big Data Analytics in Agriculture
Chapter © 2020
References
- Ahmad, Huang (2021) Big data and AI revolution in precision agriculture: survey and challenges. IEEE Access. Received 14 June 2021, accepted 30 July 2021, date of publication 3 August 2021, date of current version 12 August 2021. Digital Object Identifier. https://doi.org/10.1109/ACCESS.2021.3102227
- Alex SA, Kanavalli A (2019) Intelligent computational techniques for crops yield prediction and fertilizer management over big data environment. Int J Innov Technol Exploring Eng (IJITEE) 8(12) Google Scholar
- Alkathiri M, Jhummarwala A, Potdar MB (2019) Minería de datos geoespaciales multidimensionales en un entorno distribuido utilizando MapReduce. J Big Data 6:1–34 Google Scholar
- Amani M, Kakooei M, Moghimi A, Ghorbanian A, Ranjgar B, Mahdavi S et al (2020) Application of google earth engine cloud computing platform, sentinel imagery, and neural networks for crop mapping in Canada. Remote Sens 12(21):1–18. https://doi.org/10.3390/rs12213561ArticleGoogle Scholar
- Bal, Kayaalp (2021) Int Adv Res Eng J 05(02):309–323 ArticleGoogle Scholar
- Balducci F, Impedovo D, Pirlo G (2018) Machine learning applications on agricultural datasets for smart farm enhancement. Mach Des 6(3):38. https://doi.org/10.3390/machines6030038ArticleGoogle Scholar
- Basnet B, Bang J (2018) The state-of-the-art of knowledge-intensive agriculture: a review on applied sensing systems and data analytics. J Sens 2018 Google Scholar
- Benos L, Tagarakis AC, Dolias G, Berruto R, Kateris D, Bochtis D (2021) Machine learning in agriculture: a comprehensive updated review. Sensors 21(11):3758 ArticlePubMedPubMed CentralGoogle Scholar
- Bhat SA, Huang NF (2021) Big data and AI revolution in precision agriculture: survey and challenges. IEEE Access 9:110209–110222 ArticleGoogle Scholar
- Bhatnagar R (2018) Machine learning and big data processing: a technological perspective and review. In: Proceedings of the international conference on advanced machine learning technologies and applications. Springer, Berlin/Heidelberg, pp 468–478 Google Scholar
- Boudriki Semlali BE, El Amrani C (2021) Big data and remote sensing: a new software of ingestion. Int J Electr Comput Eng 11:1521–1530 Google Scholar
- Chergui N, Kechadi MT, McDonnell M (2020) The impact of data analytics in digital agriculture: a review. In: 2020 international multi-conference on: “Organization of Knowledge and Advanced Technologies” (OCTA). IEEE, pp 1–13 Google Scholar
- Cherkassky V, Mulier FM (2007) Learning from data: concepts, theory, and methods. Wiley Intercience, John Wiley & Sons, IEEE BookGoogle Scholar
- Cravero A, Pardo S, Galeas P, López Fenner J, Caniupán M (2022a) Data type and data sources for agricultural big data and machine learning. Sustainability 14(23):16131 ArticleGoogle Scholar
- Cravero A, Pardo S, Sepúlveda S, Muñoz L (2022b) Challenges to use machine learning in agricultural big data: a systematic literature review. Agronomy 12(3):748 ArticleGoogle Scholar
- Donzia SKY, Kim H-K (2020) Architecture design of a smart farm system based on big data appliance machine learning. In: 2020 20th international conference on computational science and its applications (ICCSA), pp 45–52. https://doi.org/10.1109/ICCSA50381.2020.00019ChapterGoogle Scholar
- Doshi Z, Nadkarni S, Agrawal R, Shah N (2018) AgroConsultant: intelligent crop recommendation system using machine learning algorithms. In: 2018 fourth international conference on computing communication control and automation (ICCUBEA), pp 1–6. https://doi.org/10.1109/ICCUBEA.2018.8697349ChapterGoogle Scholar
- Dutta R, Li C, Smith D, Das A, Aryal J (2015) Big data architecture for environmental analytics. Int Symp Environ Softw Syst:578–588 Google Scholar
- Elshawi R, Sakr S, Talia D, Trunfio P (2018) Big data systems meet machine learning challenges: towards big data science as a service. Big Data Res 14:1–11 ArticleGoogle Scholar
- Fenu G, Malloci FM (2019) An application of machine learning technique in forecasting crop disease. Paper presented at the PervasiveHealth: Pervasive Computing Technologies for Healthcare, 76–82. https://doi.org/10.1145/3372454.3372474. Retrieved from www.scopus.com
- Firdaus H, Hassan SI (2020) Unsupervised learning on healthcare survey data with particle swarm optimization. In: Machine learning with health care perspective. Springer, Berlin/Heidelberg, pp 57–89 ChapterGoogle Scholar
- Gnanasankaran N, Ramaraj E (2020) The effective yield of paddy crop in Sivaganga District-an initiative for smart farming. Int J Sci Technol Res 9:2 Google Scholar
- Gopal Maya PS (2020) Big data challenges and opportunities in agriculture. Int J Agric Environ Inf Syst 11(1) Google Scholar
- Gumma MK, Thenkabail PS, Teluguntla PG, Oliphant A, Xiong J, Giri C et al (2020) Agricultural cropland extent and areas of South Asia derived using landsat satellite 30-m time-series big-data using random forest machine learning algorithms on the google earth engine cloud. GISci Remote Sens 57(3):302–322. https://doi.org/10.1080/15481603.2019.1690780ArticleGoogle Scholar
- Haig BD (2020) Big data science: a philosophy of science perspective Google Scholar
- Hunter MC, Smith RG, Schipanski ME, Atwood LW, Mortensen DA (2017) Agriculture in 2050: recalibrating targets for sustainable intensification. Bioscience 67(4):386–391 ArticleGoogle Scholar
- Kamilaris A, Kartakoullis A, Prenafeta-Boldú FX (2017) A review on the practice of big data analysis in agriculture. Comput Electron Agric 143:23–37 ArticleGoogle Scholar
- Lassoued R, Macall DM, Smyth SJ, Phillips PWB, Hesseln H (2021) Expert insights on the impacts of, and potential for, agricultural big data. Sustainability 13:2521. https://doi.org/10.3390/su13052521ArticleGoogle Scholar
- Li H, Cheng Z, Wang H (2020) Research of agricultural big data. E3S Web Conf 214:01011. https://doi.org/10.1051/e3sconf/202021401011. EBLDM 2020 ArticleGoogle Scholar
- Liakos KG, Busato P, Moshou D, Pearson S, Bochtis D (2018) Machine learning in agriculture: a review. Sensors 18:2674. https://doi.org/10.3390/s18082674ArticlePubMedPubMed CentralGoogle Scholar
- Micheni E, Machii J, Murumba J (2022) Internet of things, big data analytics, and deep learning for sustainable precision agriculture. In: 2022 IST-Africa conference (IST-Africa). IEEE, pp 1–12 Google Scholar
- Nandi G, Sharma RK (2020) Data science fundamentals and practical approaches: understand why data science is the next. BPB Publications, Uttar Pradesh Google Scholar
- Nandyala C, Kim HK (2016) Big and meta data management for U-agriculture mobile services. Int J Softw Eng Appl IJSEIA 10:257–270 Google Scholar
- Nóbrega L, Tavares A, Cardoso A, Gonçalves P (2018) Animal monitoring based on IoT technologies. In: 2018 IoT vertical and topical summit on agriculture - Tuscany (IOT Tuscany), pp 1–5. https://doi.org/10.1109/IOT-TUSCANY.2018.8373045ChapterGoogle Scholar
- Odun-Ayo I, Ananya M, Agono F, Goddy-Worlu R (2018) Cloud computing architecture: Un análisis crítico. En Proceedings of the 2018 18th International Conference on Computational Science and Applications (ICCSA), Melbourne, Australia, 2–5 de julio de 2018 Google Scholar
- Pandya A, Odunsi O, Liu C, Cuzzocrea A, Wang J (2020) Adaptive and efficient streaming time series forecasting with lambda architecture and spark. In: Paper presented at the proceedings - 2020 IEEE international conference on big data, big data 2020, pp 5182–5190. https://doi.org/10.1109/BigData50022.2020.9377947. Retrieved from www.scopus.comChapterGoogle Scholar
- Priya R, Ramesh D (2020) ML based sustainable precision agriculture: a future generation perspective. Sustainable Comput Inf Syst 28:100439 Google Scholar
- Qiu J, Wu Q, Ding G, Xu Y, Feng S (2016) A survey of machine learning for big data processing. EURASIP J Adv Signal Process 2016:1–16 Google Scholar
- Rehman A, Liu J, Keqiu L, Mateen A, Yasin MQ (2020) Machine learning prediction analysis using IoT for smart farming. Int J Emerg Trends Eng Res 8(9):6482–6487. https://doi.org/10.30534/ijeter/2020/250892020ArticleGoogle Scholar
- Rudin C, Wagstaff KL (2014) Machine learning for science and society. Mach Learn 95:1–9 ArticleGoogle Scholar
- Saggi MK, Jain S (2022) A survey towards decision support system on smart irrigation scheduling using machine learning approaches. Arch Comput Methods Eng 29(6):4455–4478 ArticlePubMedPubMed CentralGoogle Scholar
- Saiz-Rubio V, Rovira-Más F (2020) From smart farming towards agriculture 5.0: a review on crop data management. Agronomy 10:207 ArticleGoogle Scholar
- Sarker MNI, Islam MS, Ali MA, Islam MS, Salam MA, Mahmud SH (2019) Promoting digital agriculture through big data for sustainable farm management. Int J Innov Appl Stud 25(4):1235–1240 Google Scholar
- Sassi I, Anter S, Bekkhoucha A (2019) An overview of big data and machine learning paradigms. In: Advanced intelligent systems for sustainable development (AI2SD’2018), vol 5: Advanced intelligent systems for computing sciences, pp 237–251 ChapterGoogle Scholar
- Sathiaraj D, Huang X, Chen J (2019) Predicting climate types for the continental United States using unsupervised clustering techniques. Environmetrics 30(4). https://doi.org/10.1002/env.2524
- Shelestov A, Lavreniuk M, Vasiliev V, Shumilo L, Kolotii A, Yailymov B et al (2020) Cloud approach to automated crop classification using Sentinel-1 imagery. IEEE Trans Big Data 6(3):572–582. https://doi.org/10.1109/TBDATA.2019.2940237ArticleGoogle Scholar
- Sitokonstantinou V, Drivas T, Koukos A, Papoutsis I, Kontoes C (2020) Scalable distributed random forest classification for paddy rice mapping Asian conference on remote sensing ACRS 2019. Paper presented at the 40th Asian Conference on Remote Sensing, ACRS 2019: Progress of Remote Sensing Technology for Smart Future, Retrieved from www.scopus.com
- Sukumar SR (2014) Machine learning in the big data era: are we there yet? In: Proceedings of the ACM knowledge discovery and data mining: workshop on data science for social good, New York, NY, USA, 24–17 August 2014 Google Scholar
- Šuman S, Poščić P, Gligora Marković M (2020) Big data management challenges. Int J Adv Trends Comput Sci Eng 9:717–723 ArticleGoogle Scholar
- Sundmaeker H, Verdouw C, Wolfert S, Pérez Freire L (2017) Internet of food and farm. In: Vermesan O, Friess P (eds) Digitising the industry-internet of things connecting the physical, digital and virtual worlds. River Publishers, Delft Google Scholar
- Tibbetts (2018) The frontiers of artificial intelligence. Bioscience 68(1):5–10. https://doi.org/10.1093/biosci/bix136ArticleGoogle Scholar
- Torky M, Hassanein AE (2020) Integrating block chain and the Internet of Things in precision agriculture: analysis, opportunities, and challenges. Comput Electron Agric 178:105476 ArticleGoogle Scholar
- United Nations (2019) World population prospects. United Nations Department of Economic and Social Affairs, Population Division. Retrieved November 21, 2019 from https://population.un.org/wpp/
- Wang Q, Mu Z (2022) Risk monitoring model of intelligent agriculture Internet of Things based on big data. Sustain Energy Technol Assess 53:102654 Google Scholar
- Wang X, Yang K, Liu T (2019) The implementation of a practical agricultural big data system. Paper presented at the 2019 IEEE 5th International Conference on Computer and Communications, ICCC 2019, pp 1955–1959. https://doi.org/10.1109/ICCC47050.2019.9064475. Retrieved from www.scopus.comChapterGoogle Scholar
- Weersink A, Fraser E, Pannell D, Duncan E, Rotz S (2018) Opportunities and challenges for big data in agricultural and environmental analysis. Annu Rev Resour Econ 10:19–37 ArticleGoogle Scholar
- White, Emma L.;·J. Alex Thomasson, Brent Auvermann, Newell R. Kitchen, Leland Sandy Pierson, Dana Porter, Craig Baillie, Hendrik Hamann, Gerrit Hoogenboom, Todd Janzen, Rajiv Khosla, James Lowenberg-DeBoer, Matt McIntosh, Seth Murray, Dave Osborn, Ashoo Shetty, Craig Stevenson 2021 Google Scholar
- Wolfert S, Ge L, Verdouw C, Bogaardt MJ (2017) Big data in smart farming–a review. Agric Syst 153:69–80 ArticleGoogle Scholar
- Yang C, Huang Q, Li Z, Liu K, Hu F (2017) Big data and cloud computing: innovation opportunities and challenges. Int J Digit Earth 10:13–53 ArticleGoogle Scholar
- Yang J, Liu M, Lu J, Miao Y, Hossain MA, Alhamid MF (2018) Botanical internet of things: toward smart indoor farming by connecting people, plant, data and clouds. Mob Netw Appl 23:188–202 ArticleGoogle Scholar
Author information
Authors and Affiliations
- Departamento de Ciencias de la Computación e Informática, Facultad de Ingeniería y Ciencias, Universidad de La Frontera, Temuco, Chile Ania Cravero Leal
- Ania Cravero Leal
You can also search for this author in PubMed Google Scholar
Corresponding author
Editor information
Editors and Affiliations
- Rubber Research Institute of India (formerly), Kottayam, Kerala, India P. M. Priyadarshan
- Department Agricultural Sciences, University of Helsinki, Helsinki, Finland Shri Mohan Jain
- Amity Centre for Nuclear Biotechnology, Amity University Maharashtra, Mumbai, India Suprasanna Penna
- Department of Agricultural Biotechnology, King Faisal University, Al-Ahsa, Saudi Arabia Jameel M. Al-Khayri
Rights and permissions
Copyright information
© 2024 The Author(s), under exclusive license to Springer Nature Switzerland AG
About this chapter
Cite this chapter
Leal, A.C. (2024). Data Analytics in Agriculture. In: Priyadarshan, P.M., Jain, S.M., Penna, S., Al-Khayri, J.M. (eds) Digital Agriculture. Springer, Cham. https://doi.org/10.1007/978-3-031-43548-5_17
Download citation
- DOI : https://doi.org/10.1007/978-3-031-43548-5_17
- Published : 25 January 2024
- Publisher Name : Springer, Cham
- Print ISBN : 978-3-031-43547-8
- Online ISBN : 978-3-031-43548-5
- eBook Packages : Biomedical and Life SciencesBiomedical and Life Sciences (R0)
Share this chapter
Anyone you share the following link with will be able to read this content:
Get shareable link
Sorry, a shareable link is not currently available for this article.
Copy to clipboard
Provided by the Springer Nature SharedIt content-sharing initiative